How to Ensure the Quality and Safety of AI Models in Healthcare with Independent Monitoring and Surveillance
- charlotte92502
- Jan 20, 2024
- 6 min read
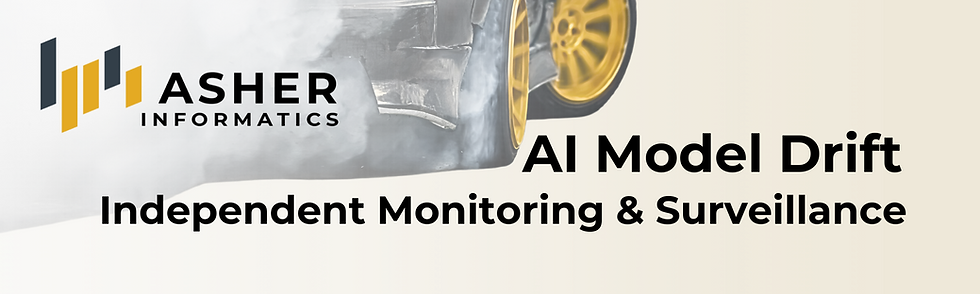
AI models are becoming more prevalent and powerful in healthcare, as they can help clinicians improve diagnosis, prognosis, treatment, and management of various health conditions and assist administrators optimize workflow processes. However, AI models are not static or perfect. They can change over time and become less accurate or reliable due to various factors. This is why monitoring and surveillance of AI models are essential to ensure that they perform as expected and deliver promised value.
But who should be responsible for monitoring and surveillance of AI models? Should it be the AI vendors who develop the models and sell the resulting applications, the AI platforms who are also financially incentivized by selling AI applications, or should it be an independent third party who can provide unbiased and objective evaluation?
In this blog post, we argue that independent monitoring and surveillance of AI models are needed, and it benefits both the AI vendors and the healthcare organizations deploying clinical AI solutions. We also explain types of drift that affect clinical AI and how to detect and mitigate them.
What is drift and why does it matter?
Drift is a term that refers to changes in the data, features, labels, concepts, or relationships that affect the performance and reliability of AI models. Drift is caused by various factors, such as:
Changes in the clinical environment or context where the model is deployed
Changes in patient behavior or preferences
Changes in data collection or processing methods throughout an AI model’s lifecycle
Changes in data characteristics, quality or availability
Changes in model parameters or architecture throughout its lifecycle
Changes in model objectives, loss-functions or assumptions
Deliberate attacks or manipulation by adversaries
Drift can have negative consequences for the AI models, such as:
Reduced diagnostic or prognostic accuracy or precision.
Decreased robustness or stability
Decreased transparency or explainability
Decreased end-user satisfaction or trust
Increased legal or ethical risks
Therefore, it is important to monitor and surveil AI models continuously over time to detect and mitigate drift and ensure that they meet the performance metrics and standards promised by the AI vendors.
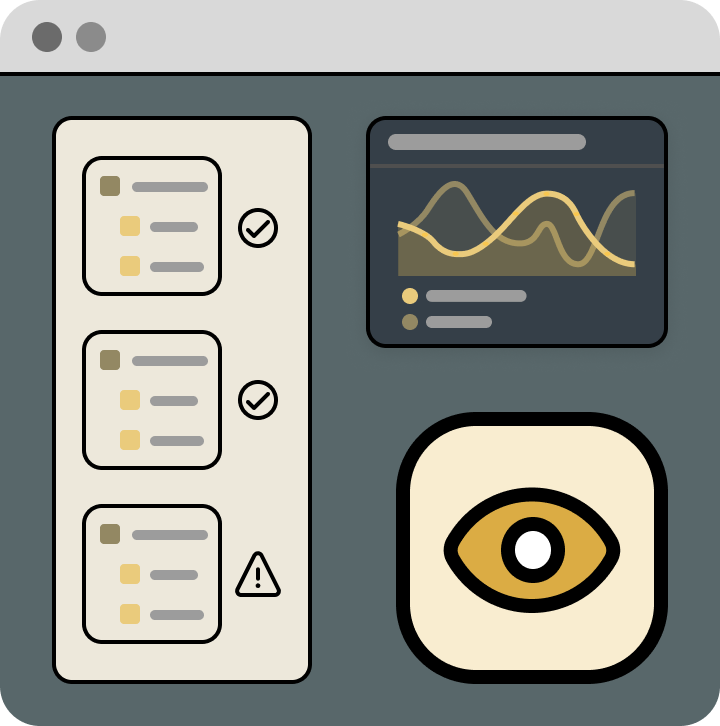
Why is independent monitoring and surveillance needed?
Monitoring and surveillance of AI models can be done by AI vendors themselves or by an independent third party. Depending upon the regulatory classification of the AI application sold, not all AI vendors may offer, have the expertise and resources to monitor and surveil their own models. Some may provide a “light review”. There may also be perverse incentives to hide or downplay data or concept drift and its impacts. Here are some of the concerns if healthcare systems are relying on AI vendors to self-monitor:
AI vendor might overstate or exaggerate the performance of their applications in a specific healthcare setting and practice
Non-regulated AI applications may not have rigorous risk assessments performed prior to deployment
From a user’s perspective, take a long time to update or change a suboptimal AI model’s performance
End users, administrators, and clinicians, need evidence and information regrading an AI application’s performance. We believe insights are least biased and reliable if they are delivered from someone other than the developer of the AI models and application. Independent monitoring and surveillance can provide more credible and objective evaluation of AI models and their impacts. When managing and overseeing the use of clinical AI application in their clinical environments, healthcare organizations should expect high confidence that their investment in clinical AI tools are providing safe and effective benefits to their patients, staff, and stakeholders. A trusted, outsourced partner can provide the following, important capabilities in an independent fashion:
Verify or validate the performance or benefits of the models
Identify or expose the limitations or risks of the models
Use or recommend the best practices or standards to measure and report AI outputs, accuracy, and benefits to the healthcare organization
Suggest or require updates or improvements to deployed AI models
Enforce or ensure accountability or responsibility for AI applications’ use and comprehension
Improve change management, increase confidence, and improve trust with the end user.
Finally, independent monitoring and surveillance can benefit both AI vendors and the healthcare organizations by:
Improving the quality and safety of the models and application
Enhancing the confidence and trust in the AI
Increasing the adoption and satisfaction of the end-users
Reducing the legal and ethical issues of the models
Promoting innovation and a culture of learning and continuous improvement
How to monitor and surveil AI models for drift?
There are different types of drift that can affect AI models and different ways to monitor and surveil them. Here is a list of the most common types of drift and some of the methods to detect and mitigate them:
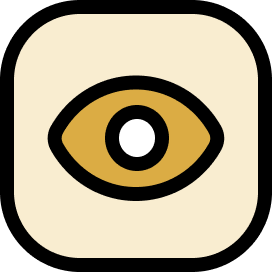
Concept drift: This occurs when the underlying relationship between the input features and the target variable changes over time. For example, the risk factors or outcomes of a disease may depend on different factors in different populations or settings. To monitor concept drift, one can regularly assess the model performance on new data and use statistical tests, monitoring metrics, and clinical expertise to detect shifts in the target concept. To mitigate concept drift, one can update or retrain the model with new data or use online or adaptive learning methods to adjust the model dynamically.
Data drift: This occurs when the statistical properties of the input data distribution change over time. For example, the distribution of patient demographics or clinical variables may change due to changes in the population or practice. To monitor data drift, one can compare the distribution of incoming data to the distribution of the training data and use statistical measures, such as Kolmogorov-Smirnov tests or clinical metrics, to detect data drift. To mitigate data drift, one can update or retrain the model with new data or use data augmentation or transformation methods to align the data distributions.
Feature drift: This occurs when the characteristics or values of specific features used by the model change over time. For example, the meaning or usage of a clinical term or code may change due to changes in the guidelines or standards. To monitor feature drift, one can regularly inspect the distribution and statistics of individual features and identify shifts or anomalies in feature values that may impact model performance. To mitigate feature drift, one can update or retrain the model with new data or use feature engineering or selection methods to modify or replace the features.
Label drift: This occurs when the distribution of target variable labels change over time. For example, the definition or diagnosis of a disease may change due to new research or evidence. To monitor label drift, one can check the distribution of labels in the training and test datasets and use statistical tests or clinical knowledge to detect label drift. To mitigate label drift, one can update or retrain the model with new data or use label smoothing or correction methods to adjust the labels.
Covariate shift: This occurs when the distribution of input features change over time but not necessarily the relationship between features and the target variable. For example, the distribution of patient symptoms or signs may change due to changes in the screening or testing methods. To monitor covariate shift, one can use the same methods as data drift. To mitigate covariate shift, one can use techniques like importance weighting or re-weighting to account for changes in feature distribution without retraining the entire model.
Population drift: This occurs when the characteristics of the overall population being modeled change over time. For example, the population of a hospital or a region may change due to changes in the admission or referral patterns. To monitor population drift, one can assess whether the demographics or characteristics of the population have changed and use statistical tests or clinical knowledge to detect population drift. To mitigate population drift, one can update or retrain the model with new data or use stratified sampling or weighting methods to reflect the current population.
Temporal drift: This occurs when patterns or relationships change over time. For example, the behavior or preferences of patients or clinicians may change due to changes in the trends or seasonality. To monitor temporal drift, one can analyze time-series data to detect trends, seasonality, or shifts in behavior and use statistical tests or clinical knowledge to detect temporal drift. To mitigate temporal drift, one can update or retrain the model with new data or use time-series analysis or forecasting methods to capture evolving patterns.
Adversarial drift: This occurs when adversaries deliberately attempt to manipulate or deceive the model by changing the input data or the model itself. For example, hackers may try to fool a diagnostic system by altering the images or records. To monitor adversarial drift, one can employ techniques like adversarial training or anomaly detection to detect and mitigate adversarial attempts to induce drift. To mitigate adversarial drift, one can update or retrain the model with new data or use robust or secure learning methods to protect the model from attacks.
Conclusion
As the number of clinical AI models being developed grows, healthcare organizations will be utilizing multiple models that are both third-party and homegrown. There are also high-value scenarios in which ensembles of several models chained together (potentially sourced from different vendors or built internally). As the diffusion of AI into the clinic increases, the complexity of their deployments will make it even more important that healthcare organizations develop and maintain evergreen processes and systems to ensure their machine intelligence solutions are working as intended, hoped, and are providing maximum economic ROI and clinical benefits to patients and providers. Independent AI monitoring and performance evaluation services will be a key recipe to realizing that future.